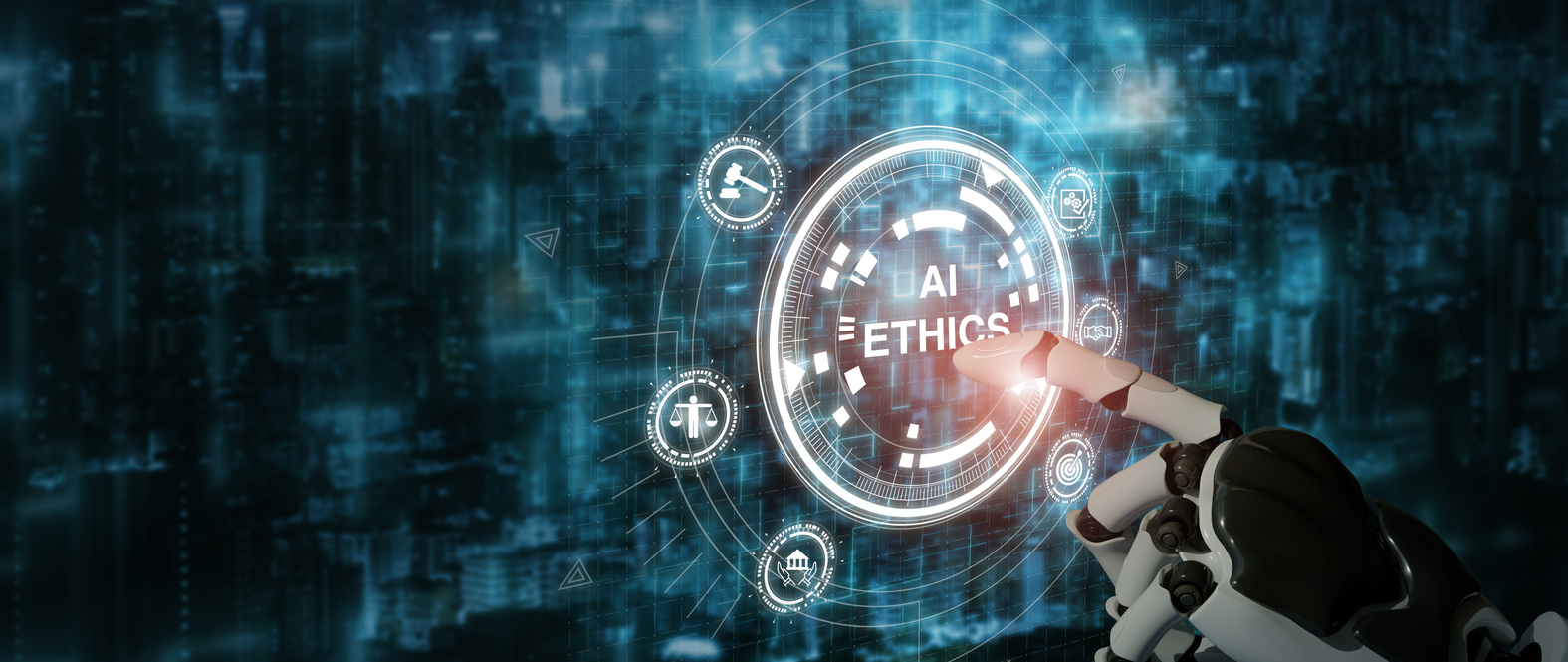
Artificial intelligence (AI) and machine learning (ML) are revolutionizing decision-making across industries—from healthcare and finance to law enforcement and social media. These technologies promise speed, efficiency, and objectivity, offering data-driven insights that often surpass human capabilities. However, as algorithms become more deeply embedded in everyday decisions, concerns about ethics, transparency, and accountability have grown. The challenge lies in ensuring that AI serves humanity fairly, responsibly, and without unintended harm.
In this article, we explore the ethical dimensions of AI and machine learning in decision-making, the risks and benefits, and how society can balance innovation with integrity.
1. Understanding the Role of AI in Decision-Making
AI and ML systems are designed to analyze data, identify patterns, and make predictions or recommendations. Their applications are wide-ranging:
Key Use Cases:
- Healthcare: Diagnosing diseases, recommending treatments
- Finance: Credit scoring, fraud detection
- Criminal Justice: Predictive policing, parole decisions
- Hiring: Resume screening, candidate ranking
- Social Media: Content moderation, feed personalization
While these tools enhance efficiency, they also raise important questions about fairness and control.
2. The Promise of Ethical AI
When used responsibly, AI can promote fairness, reduce bias, and improve decision-making.
Potential Benefits:
- Eliminating human bias: By basing decisions on data rather than emotion or prejudice
- Scalability: Analyzing vast datasets quickly and consistently
- Accessibility: Enhancing services for underserved populations (e.g., rural telemedicine)
- Objectivity: Providing consistent outcomes in rule-based environments
These benefits hinge on high-quality data, transparent algorithms, and proper oversight.
3. Key Ethical Concerns in AI-Driven Decisions
Despite its potential, AI can also perpetuate or amplify existing inequalities.
Major Ethical Issues:
- Bias and Discrimination: Algorithms can inherit bias from historical or unrepresentative data.
- Lack of Transparency: “Black box” models make it difficult to understand how decisions are made.
- Loss of Accountability: Who is responsible when AI makes a harmful or unfair choice?
- Surveillance and Privacy: Overcollection and misuse of personal data raise civil liberty concerns.
- Autonomy and Consent: Users may not fully understand or agree to algorithmic influence.
Ethical AI development must directly address these challenges.
4. Bias in AI: A Deep-Rooted Issue
Bias is often embedded in the training data used to develop ML models.
Examples:
- Facial recognition systems have shown higher error rates for people of color.
- Hiring algorithms may replicate past hiring biases, such as gender discrimination.
- Predictive policing tools have disproportionately targeted minority neighborhoods.
These biases can lead to unfair outcomes unless datasets are carefully curated and monitored.
5. Transparency and Explainability
Understanding how AI reaches its conclusions is critical for trust.
Best Practices:
- Use interpretable models or apply explainable AI (XAI) techniques
- Maintain documentation and model cards that describe algorithm behavior
- Provide users with clear explanations and justifications for AI-driven decisions
Transparency builds confidence and enables informed oversight.
6. Accountability and Responsibility
When AI systems make decisions, assigning responsibility becomes complex.
Considerations:
- Developers and data scientists must ensure ethical design and testing
- Organizations using AI should establish clear lines of accountability
- Policymakers need to set legal frameworks for liability
- Users should be empowered to challenge or appeal automated decisions
A shared responsibility model can help distribute roles more equitably.
7. The Privacy Paradox
AI systems often rely on extensive personal data.
Ethical Questions:
- What data is collected, and how is it used?
- Are users aware of and consenting to data usage?
- How is sensitive data protected from misuse?
Regulations like GDPR and CCPA provide frameworks, but enforcement and global consistency remain a challenge.
8. Consent and User Autonomy
Many users are unaware when they are interacting with or being influenced by AI.
To Promote Ethical Use:
- Inform users when AI is making or assisting with decisions
- Offer opt-out options or human oversight where possible
- Ensure that consent is informed, not buried in fine print
Transparency empowers individuals to make informed choices.
9. Ethical Design and Development Practices
Building ethics into AI systems requires intentional design.
Guidelines for Developers:
- Use diverse datasets and test for disparate impacts
- Engage ethicists and multidisciplinary teams in model design
- Apply fairness metrics and perform algorithmic audits
- Prioritize safety, security, and inclusivity in product roadmaps
Responsible development is proactive, not reactive.
10. The Role of Regulation and Governance
Legal frameworks are essential for ensuring ethical use of AI.
Key Actions:
- Define standards for algorithmic fairness and transparency
- Require impact assessments for high-risk applications
- Promote open-source and independent auditing of AI tools
- Support public education on AI rights and protections
Governments, institutions, and industry bodies must collaborate to create enforceable policies.
11. The Importance of Human Oversight
AI should complement—not replace—human judgment.
Examples of Oversight:
- Human-in-the-loop (HITL) systems that allow manual review
- Escalation protocols for edge cases
- Periodic audits and performance evaluations
Maintaining a human touch ensures that ethics remain central to decision-making.
12. The Future of Ethical AI
As AI continues to evolve, ethical considerations must keep pace.
Emerging Trends:
- Development of “AI ethics by design” frameworks
- Growth of ethics-focused roles within tech companies
- International cooperation on standards and regulation
- Broader public discourse on algorithmic accountability
The ethical future of AI depends on continuous dialogue, vigilance, and adaptation.
AI and machine learning are reshaping how decisions are made, offering enormous potential for efficiency and innovation. But with this power comes responsibility. Ensuring ethical AI requires transparency, fairness, accountability, and respect for human rights. By embracing these principles from design to deployment, we can harness the benefits of AI while safeguarding the values that define a just and equitable society.